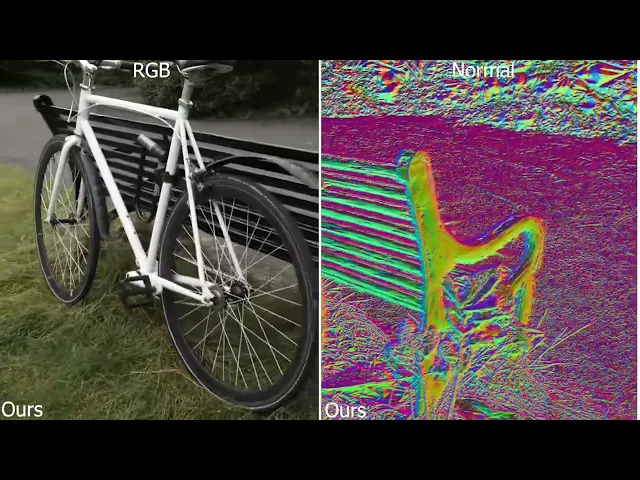

Michael Rubloff
May 30, 2024
Over the last month, we have repeatedly seen methods that have been exploring Gaussian Splatting to increase fidelity and surface reconstruction. These have ranged from 2DGS, to Surfels, and more. Today, we’re looking at a slightly different take on the problem with AtomGS.
There are two main steps to how AtomGS works: through Atomized Proliferation and Geometry-Guided Optimization.
Atomized Proliferation constrains ellipsoid Gaussians of various sizes into uniformly-sized Atom Gaussians. It emphasizes the densification of smaller Gaussians, filling gaps in the geometry and ensuring detailed scene representation. After the Atomized Proliferation stage, the final prune stage enables Gaussians to adjust their scales based on the complexity of the scenes. During this refinement process, Gaussians that capture intricate high-frequency details are preserved, while those representing broad and smooth surfaces are merged.
By doing so, it places greater emphasis on densification in accordance with scene details, significantly enhancing the representation of areas with fine features.
Secondly the Geometry-Guided Optimization introduces an Edge-Aware Normal Loss that combines the curvature map and edge map to maintain geometric accuracy. The normal map represents surface orientations, while the curvature map indicates surface normal variability. The edge map ensures high-frequency details are preserved by excluding them from excessive smoothing. This balanced approach results in enhanced rendering quality and accurate geometric reconstruction.
Similar to other methods, AtomGS will train on upper end consumer cards, with their own experiments being completed on a single 4090.
The authors mention that their method can benefit from a stronger pruning strategy and coincidentally one appears to have recently emerged.
For more interactive demos and detailed insights, visit the AtomGS project website: AtomGS Project. Their code has additionally been published for people to try out for themselves!