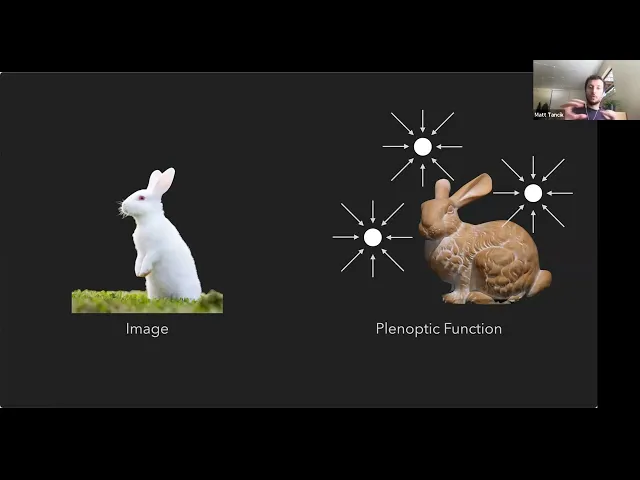

Michael Rubloff
May 10, 2023
Matt Tancik, Co-Founder of Nerfstudio and the original NeRF paper, joined the class of MIT MAS.S61.
The lecture gives an amazing overview of NeRF, the evolution of art, and how NeRFs might evolve going forwards. I highly recommend giving it a listen or reading through the transcript below. He does a great job showcasing how NeRFs can be applied in so many more ways than how they are being thought of now.
Professor: Welcome back everyone to our interviewers class week nine about synthetic realities. This is a really really interesting talk as well, you have Matthew Tancik speaking today to us he is a PhD student at UC Berkeley is one of the co-authors of the original NeRF paper a field that exploded two and a half years or something ago. It's every week I see something new, so this is all we're speaking to the origin of the explosions so to say. Matthew is also an MIT media lab alum and so we're super happy to have you back in the media lab and the stage is yours.
Matt Tancik: Thanks. Yes, so today I'll be talking about NeRFs and throughout my presentation, feel free to just chime in, ask questions. I'll try to keep an eye on the chat. To get started I want to kind of start in maybe a slightly different place, which is to sort of pose two questions, which will sort of come up throughout this talk, which is what is the role of an artist and also what is the role of technology particularly in terms of art. And I think in the previous q&a with stability folks like some of these questions were sort of discussed that's I think that's a good context.
So first I'm going to give a very condensed biased view of how I've kind of view 2D art has evolved over time. If you go all the way back in time, thousands of years ago, you have these cave paintings. And I think it's interesting to ask the person who painted this, did they paint this with the like a story in mind? Were they trying to replicate what they saw around them? What was the purpose? Now obviously we can't answer it in this particular case, but then as you sort of fast forward through history, you get to say the Renaissance period, where we've developed our tools, we've developed our skills, and what we start to see is more paintings that try to mimic the world around us being photorealistic. But at the same time obviously, Francois (Desportes), who painted this painting did not see three rabbits posed like this. So they're really trying to tell a story and I think what's interesting here is quite often to be an artist at this time period it was highly conditioned on being able to actually produce this type of art having the technical skill to make these very intricate paintings and so in that sense it very much restricted who could be an artist. Because if you had a brilliant idea you knew what you wanted to see but you didn't have the skills to do it, you were sort of out of luck. And I think this has evolved a lot over the last hundred years or so really with the advent of photography. Because now there's no longer as much of a need to create photorealistic paintings because you could always just pull out your camera and take a photo. So as a result you kind of see art take a slightly different direction. If you just want to tell a story you can draw a cartoon you don't care about the photo realism anymore. If you do care about the photorealism, it's more about how you set up your photos how you maybe create your video less so about the actual photorealism of the content.
So why am I mentioning any of all this? Let's consider what's happening in the 3D domain. So here's three different or four different applications of where people use 3D models. In the top left you might use it for gaming and in many cases you want a photorealistic environment that you explore. In the top right you might use 3D content for simulation, for robotics; in this case this is a self-driving car company that wants to simulate how a car might drive around. Maybe you just want to document the world around you in which case you want it to be accurate. Or maybe what you're trying to capture just doesn't exist in real life and so you still have to create it in 3D, but you want it to be something that looks feasible and this is particularly prevalent in like the field of VFX. So where do these 3D models come from? Well most of the time it's going to be some 3D artist sitting behind a computer working with programs like Blender to create these models and it's a very time intensive process. This time lapse I'm showing you here is 30 minutes and it's also sped up but ultimately the artist does achieve making this sort of realistic looking toy model that maybe you can read a story in it, but a lot of effort went into creating this. You can even quantify that effort by just assigning the value to each asset in the scene.
So there's a 3D artist Andrew price who did it for a modern video game and what you see is you end up spending potentially thousands of dollars to produce 3D models of things like trash and so you have to take a step back now and ask what is the point of the artist here like what is a 3D artist is a 3D artist a person who can mimic the reality or is it the person who's telling the story setting up the environment sort of creating the situation that they want people to experience. And so this all sort of leads back to sort of this idea that maybe 3D art is where the Renaissance painters were at that time, where in order to operate in that space you had to have all this technical skill; and if that's the case where do we want to be? I'd argue we'd want to be in a place where we have technology to do maybe some of the more laborious aspects of it creating the photorealism and so in that sense what is the 3D equivalent of a camera?
So that's sort of the purpose of this talk and one of the motivations as to why we might want these 3D technologies. Not necessarily to replace artists, but to make it so that the role of the artist is more in the creative aspects of 3D generation, less so in the more tedious replication of the 3D world around us.
So the first question we have to ask is or rather specify is like what what is our ultimate goal? The ultimate goal of a photograph is just to be a 2d projection of the world. That's fairly easy to define. What is it in the case of 3D? So the thing that we want to recover is something called the plenoptic function. what the plenoptic function is, is from any point in space if I look in any direction, I want to know what color I would see. If I had access to this plenoptic function, then I would be able to explore this scene and it will look correct regardless of how I move around in it. So we need to start from something. An image starts from some hardware that takes a photo and produces it. how do we start this creation process of a plenoptic function? We're going to make the assumption that we're going to have a set of images of the scene and more specifically we're going to know where those images were taken with that camera.
Okay so we know what we want to ultimately accomplish. The first thing we have to ask is how do we represent this plenoptic function? There's a variety of different ways to represent 3D objects and so let's go into a few different options and discuss the pros and cons and why are why not they may make sense for this particular application.
By far and away the most common 3D representation is a mesh representation. So you describe an object with a bunch of polygons, often triangles, to describe the scene and this is what you see in video games in movies and phone apps it's very common and highly optimized. So if I wanted to create an algorithm that creates a mesh, given some information, I need to have some method to transform and actually generate that mesh. And so one common approach is to do something like a gradient based optimization and the idea here is if you start with some initial guess and you have the target that you're trying to fit and you're going to compare that target with your initial guess, compute some form of loss how far off you are and then update your initial guess to better match that target. So you can repeat this process over and over again until you eventually reach the ultimate geometry that you're interested in.
Now it works fine in this case but very quickly you realize that it's not quite that easy all the time. What happens if you have for example multiple objects? Now you might need some way to split up your initial guess into multiple chunks. What if your target is just a different topology? How do you turn a sphere into a donut? You might need ways to modify the topology of your initial guess. What happens if your target has areas that are highly detailed and you just don't have that much that many vertices or points in your initial guess? Now you might need ways to move them around or add new ones to the scene. So these challenges are all solvable and there's a lot of research in the space and people have made a lot of progress. My main point in raising them is that when working with these types of representations, the optimization for how you actually program these algorithms ends up being quite tricky. So what other representations may we consider?
One option is a voxel representation. So classically the most famous voxel representation is something like Minecraft, but the idea is you just have a bunch of boxes that describe your 3D object. If we do that exact same gradient based optimization but in this voxel grid format, you quickly see that it has different side effects. So similar to before we compute a loss and we can keep making updates until we recover our target geometry. And what's cool about the voxel representation, is if the topology or the the scene changes a lot, it just naturally still works.
Now there's one fairly obvious downside to voxels which is one of resolution. So if I have a low resolution voxel grid, instead of recovering say a triangle or pyramid, you end up getting this sort of stepped pyramid. And so in practice, when using voxel grids, you need to work at higher resolutions. I mean it's the same thing with images, but the only challenges as you go higher and higher resolution you're because you're working in 3D that third dimension starts growing quite a bit and very quickly, you're unable to fit it in memory or operate on it with whatever computational tools you want to. So, and it's worth noting that in comparison to a mesh a mesh can describe the same thing, but often with a lot less information.
So we're in this sort of situation where meshes are easy to sorry meshes have small memory footprints, but they're very hard to optimize, whereas voxel representations are kind of the opposite. So what do we do? Is there is there any other alternative? Well it might seem a little bit out there at first but maybe we use something like an implicit function so as a reminder from I don't know, middle school or high school, you can represent 3D objects with just equations. So a sphere can be x² + y² + Z² = 1 and now what's cool about this representation, is if I zoom in on in on the sphere it's a perfect description of a sphere.
I can zoom in forever and it's still 100 accurate. In addition to that, the description of it this equation is extremely compact it's hard to think of a way to more compactly describe what a sphere is. Okay well a sphere is fairly simple. Can we do more complex objects? Sure if we just start tiling multiple equations together, we can now start to describe more complex objects. For example, someone sat down and managed to figure out a bunch of equations to make a rabbit. Now this is only scalable to some extent. Like if I wanted to now reproduce the leaves in a tree, there's no way a human would be able to sit down and actually write out all the equations to do that. So is there a way that we can essentially use technology to do that for us? And it just so happens that we've been developing this technology for quite some time now which is basically neural nets, because all a neural network is is a bunch of equations. So if we set it up in a way that we can update those equations using gradient descent, we can describe these complex 3D objects.
So how might you describe a 3D object with a neural network? Well one option is you can imagine having a 3D XYZ location that gets fed into a network and then the network predicts whether or not that location is occupied. If I densely query that 3D volume, I can now get a 3D representation of the scene. And so this is maybe a little bit weird way to think of 3D geometry because what we're ultimately doing is we're storing that geometry in the weights of a neural network. There's different variants for how we actually store that information. We could have it predict occupancy; we could have it predict the distance to a surface maybe it includes other information like color, but ultimately this information is stored in this like compressed format as the neural network. And so NeRF just extends this to a slightly different way of what you pass in and and take out of the network. So in the NeRF case, we're going to again feed in this XYZ location in space, but we're also going to say what direction we're looking at that point and then the output to this network is going to be the color at that location and then how much stuff or the density of that point in space.
So if I want to render a scene now, what I'll do is given some target camera I'm going to shoot a ray out into the scene and I'm going to take samples along this ray and for each of these samples it's going to be corresponding. It's going to correspond to a single XYZ value and the viewing direction Theta Phi that's correlated with that camera ray. Those values will be passed into this network, which will then predict the color and density. So we can use that to update the sample and we're going to do that for all the samples along this ray.
Now that we have all these samples, we're going to composite them together using volumetric rendering and compute a single color for that specific raise pixel. And if this is a training image, we can compare it to the ground truth value and use that as a loss to update this neural network. But once we have this model, we can now go anywhere in the scene and render it out using the same approach since the scene is now stored as the weights of the neural network.
So we can apply this to real images. Start take a few images train this neural network and now we can move around and see the reconstruction of the scene. And since we're feeding in the direction that we're looking at each point, we're actually able to capture changes in appearance as you move around the scene. So these are the view dependent effects non-lumberation effects such as the specularity or the shininess of the table or the screen. Additionally this network is predicting color and density at every point of space, so that density effectively tells us is there something there and it tells us the 3D geometry and this allows us to extract the 3D information in the scene which can be used for a variety of things such as inserting objects into the scene that are properly occluded. You could also then take this geometry and extract it into more traditional formats like meshes or voxel grids. So that's sort of like the high level overview of what NeRF is and the first thing to note is it has a lot of assumptions. It assumes that you know exactly where the cameras are in the scene, it assumes that the scene hasn't changed at all, and what I mean by that is the lighting hasn't changed between each image you've taken. There hasn't been objects moving around, a person hasn't walked across, there's a lot of these assumptions and as a result when you actually try to bring these out into the real world, they typically —these NeRF models just fail. And so over the last few years, people have started coming up with a bunch of tricks to account for these different assumptions to relax them and make these models more robust.
For piling these tricks onto NeRF you can optimize cameras there's a long list of them I'm not really going to go through it but the point is once you start adding these tricks together you can start bringing them out into the real world and this is what we did in this project called Block NeRF where we this was with Waymo a self-driving car company where they had a lot of data of San Francisco and so they were curious if you could then take all this data learn these NeRFs from it to essentially reconstruct large portions of the city, which can then be used for simulation efforts and other parts of the the robotic pipelines and one thing that's kind of cool that is specific to these types of representations, is I talk about feeding in the location and the viewing direction as the input to these neural networks, but these neural networks are flexible. I could feed in additional information if I wanted to. So for example I could feed in time of day or maybe weather and then when I render, I just choose which of these parameters I want to render with and this allows us to do these shifts of lighting and appearance.
So I'll play a couple more of these Block-NeRF videos, but while they're playing maybe I can take a quick pause and see if there's any questions about just NeRFs in general.
Student:
Okay yeah how does the coordinate system work with NeRF, because you're giving yeah like with these for example where yeah how is the coordinate system set up and it's it seems like it's estimating this city-wide continuous density function, but it yeah how does that work? Curious about that.
Matt Tancik: Yeah so the coordinate system and how like the scene scale and all that comes from ultimately it comes from the the cameras that you take these images with. Because we make the assumption that we know exactly where these cameras were located, we used other algorithms to essentially find their poses and so everything is relative to that.
Student: Okay so oh it's it's literally viewer. Okay. it's the coordinate system based on the viewer. The observer.
Matt Tancik: Yeah. Yeah.
Student: Okay Cool.
Matt Tancik: I mean I guess it is a world coordinate system.
Professor: I have one question, Matt. So you said earlier you could feed all kinds of things into like density color and so on. Something that just doesn't quite match in my mind but somehow feels like it could fit into this is the world of stimulation and you have not the density as of is there something or not, but maybe you know material. Is it steel? Is it fluid? is it parts of them? Like the kind of you know I can't really grasp it but it feels to me like there's a there's a whole open world in which you could think about simulation from a totally different perspective.
Matt Tancik: Yeah. I guess two comments there. So you're right. You can change what the outputs of these networks are. Density and color was just sort of an easy option and there's been a lot of follow-up work that predicts instead of that it predicts albedo, and specularity, roughness, lighting, these other properties to act more like a traditional graph extension so so that's one direction you can go. And then another direction which I'll talk about at the end of this talk is actually having it predict something that isn't physical anymore but more semantic and the possibilities of predicting these semantics like what what that ultimately allows you to do. So I'll talk a little bit more about that later foreign.
I'll continue so NeRF came out roughly three years ago and since then there's been a lot of follow-ups. This is a GitHub repo that tracks the number of these follow-ups. What's cool is a lot of these methods start fixing many of the issues that you have with the original NeRF method. And that's kind of what we did in the the Block NeRF Waymo paper. We combine a variety of these methods together, but there's a challenge in that in that each of these methods are often implemented in their own code bases, so actually bringing them all in takes a fair bit fair amount of effort and it it's kind of annoying that you have to do all this work before you even get started with what you're interested in accomplishing.
So that's what really motivated us to start this framework which we call nerfstudio to help with this development and so this was work done with a group of us at UC Berkeley and Angjoo Kanazawa's lab and what's cool is since we've released it in October, the community has also helped out quite a bit with the development of their studio. In fact there's people at MIT Labs who have also contributed to this project so at a very high level we had three main goals:
The first being that we wanted it to be easy to use, so NeRFs are a very research topic a research heavy topic and as a result it can be a little bit intimidating for someone who's not in the space to get started whether it's an artist or maybe a researcher in a different field such as robotics or VR. And so we wanted to try to make it as easy as possible. You still have to know a little bit of like what Python is and how to git clone, but after that you don't really need to know NeRF specifics in order to use this framework.
The second thing is we wanted it to be easy to develop so let's say you are interested in these methods we've designed the framework to be python-based and modular so that you can piece together things without really knowing a lot of the lower level Cuda C++ type optimizations that are often necessary to get these methods to work quickly.
And then finally, we wanted to provide a area for people to learn more about NeRFs since the field is moving rather quickly having both blog post style like descriptions of different methods and also community efforts in order to learn NeRFs, we think is a valuable contribution.
So the framework at a high level consists of a few components. First there's the input pipelines. How do you get the data into these models? The second is these actual methods. How you combine these things together in order to create some method that can produce a NeRF? Then we've got a web viewer that allows you to visualize these NeRFs and also visualize them as they train to get an idea of whether or not whatever method you're using actually makes sense for that data. And then finally once you have these NeRFs, we have a variety of tools that let you export them into other formats for downstream applications.
So I'll sort of walk through each of these in turn the first starting with these different data pipelines as your question mentioned earlier like what are the coordinate systems? I think there's a lot of questions on how you actually get the data into the format needed to actually compute these NeRFs and it often ends up being a bottleneck for many users. There's some open source software such as COLMAP that lets you do this, but it is tricky so we've tried to make some scripts to make a little bit easier. But then we've also provided support for web or phone apps like Polycam, Record3D and KIRI Engine that make it very easy to just capture phone data with your phone and then import it into our pipeline. Professionals, especially in the VFX industry, also use tools like Metashape and Reality Capture, which are desktop-based applications, which we've also added support for in our studio.
On the coding side, we've split up the NeRF into a variety of different components to try to make a little bit more modular. So I'm not going to go too much into details, but you can kind of think of it as sort of three high-level components to a NeRF. One is how you make those samples along the ray. The second is how you store that information into this neural network. And the third is how you take that those results and then composite them into like the final colors and so for each of these. There's a variety of different options proposed in different papers and you can kind of mix and match them for your particular application.
And this list is constantly growing as more papers are coming out and more people are implementing new methods into the repository, but as sort of a case study we developed this model which we call nerfacto this art de facto NeRFmodel where we had the goal of making it fast to train fairly high quality. But then also not super reliant on like huge GPUs it can work on some less intense systems. You still need GPUs but not state-of-the-art ones and what's cool here the the actual arrangement of these modules is not important, but the cool part is that these modules come from different papers and they can just piece be pieced together almost like lego blocks to accomplish this set of constraints that we wanted in the in the nerfacto model.
Okay so that was sort of the development side, then there's the viewer side where we have this viewer you can view your NeRFs. You can kind of see where your training images were that you used to construct this NeRF. You can create camera paths you can create trajectories and once you have these trajectories you can then go in and actually render out videos from these NeRF models. This is something that like at least in for me in the past has always been really tricky. It often involves a lot of trial and error using colabs trying to build these things so actually being able to visualize and mess around with this in a 3D viewer I think is quite useful. We have other features in the viewer you can sort of investigate your NeRF by like cropping it to kind of get an idea of where it assigns density. You can specify to output other things than just RGB. It can output depth, potentially other outputs, which can help you debug your models if you're working on developing them. And then one thing that we actually added just recently, is the ability to actually modify what is in the viewer to be specific to your specific needs in your model. So you can add text boxes, text box-check boxes, drop downs, Etc. And these parameters only are added with only a single line of code and you can access them and do whatever you want with them in your actual code. So for example, you could turn off and on a loss function while you're training just to see what would happen. Or you can maybe change the exposure of your input image. Or you could really do anything; it's it's maybe a little bit too flexible in that you can like break everything if you wanted to, but we at least give people the option to if they want to.
Okay so that's on the development side now that you have these models what do you do with them. So you could try to export them to use in downstream applications, so we have the ability to export point clouds. You can also export meshes this conversion of NeRF to other formats such as meshes. It is still very much an ongoing area of research what we've implemented in nerfstudio is a very naive approach and one thing we hope to do going forward is to improve upon these approaches and use more state-of-the-art methods in the literature. You can also just export videos. This is how you get the actual high quality NeRF renders and we have a variety of tools that let you create camera paths and actually like play with things like changing the focal length to create dolly zoom effects and other effects that would be impossible to actually create in real life. And to help with that we've made a Blender add-on, which allows you to actually create the paths in Blender so that you can render out your background NeRF. And if you have 3D models in Blender you can kind of composite them together to create these VFX style effects.
All right, this is someone in the community for example who took our Blender plug-in and just has been doing lots of cool explorations with nerfstudio. there's also other efforts so this is actually a company that has built called Volinga that has built this interface with Unreal Engine so that you can train your model in nerfstudio but then actually just open it up in Unreal and explore it and basically treat it like a video game asset.
So a few other notes we have a colab so people don't have GPUs they can get started you can upload your own data and train these models. It's worth noting that colab is somewhat slow, so it's not the best user experience, but it's at least free. Colab also seems to change their backend quite often, so it's we're always updating it trying to keep it up to date, but sometimes it's it doesn't work when colab makes bigger changes. We also have documentation where we're always adding to it. We include interactive guides to explain some of the more technical details in addition to guides that explain different models that we have implemented and that others in the community have implemented to get an idea of how they work in a way that you don't necessarily have to go to these research papers and read a lot of dense text but maybe a little bit more user friendly.
And there's also a Discord that we've been maintaining where people can post their own renders, people can ask questions about NeRFs research-based, just yeah whatever you want about NeRFs. You can kind of come here and ask questions and learn more about them. And speaking of the community, I think one of the fun things about doing this project is actually seeing this research that I've been a part of for the last few years making it out into the hands of other people to see what other people actually do with it. So I'll just play through some videos and as these are playing I can pause for questions.
Student: I'm not in this class I just came today. So I was looking at on the site like the NeRF to NeRF and a lot of the like I guess NeRF editing stuff like you were saying like add weather and it seems like at least in NeRF to NeRF it's editing the actual images versus where's the camera oh there it is. Editing the actual images and not the point cloud. Is that the way that you're thinking about it and that everyone's thinking about it? Or is there also this idea of like a point cloud latent space that people are editing? Does that make sense?
Matt Tancik: Yeah. Actually the next thing I'm going to talk about is NeRF to NeRF but as just a quick preview like I think it is very much an open question of as you start doing these editings like which domain should you be editing in. Do you edit in the actual NeRF space? Do you export it into mesh or some other format before you do editing? Do you do the editing before you even start this? I don't think I have a great answer for that yet and I think the community as a whole is still trying to figure that out.
Student: Cool thanks.
Matt Tancik: Yeah.
Student: How does this work have you are you familiar with the literature on like surface reconstruction? I work with a lot of small features on the ground from aerial photography and I'm looking to reconstruct the surface and go. And it's not mysterious if you're familiar with the effectiveness for that those types of applications?
Matt Tancik: I mean people have definitely explored it. I haven't played as much in that particular domain, so I'm by no means an expert. In general the way that these NeRF models work is they highly rely on having viewpoints that are at strong angles from each other in order to better triangulate and figure out how these scenes work. And so in practice, the more stronger angles you get the better reconstructions you get. So what I've seen is like when people are working with satellite imagery for example, sometimes you get other artifacts that you then have to account for where it'll want to place this NeRF geometry right in front of each camera because from those viewpoints it looks fine. And there's nothing to really say that that's incorrect. Versus if you have one like this car case, if you try to play something right in front of the camera the camera that's viewing the car from the other angle will see suddenly this like blob floating in the the space and so it's able to get rid of it. So as a result in cases yeah like satellite imagery, you have to do additional priors on the scene where you say for example, don't add anything closer than a kilometer from the camera and that's how you get around some of these issues. So it's definitely possible and people are working in the space, but the exact priorities you use tend to be a bit different, so ultimately it's kind of tricky to have like one solution that fits every situation.
Student: Thank you.
Student: it's very similar to that basically like yeah some of the work that me and some of the people are working on through this movie is again service reconstruction for like aerial surveying of like geological sites and some of the trickiness there is you actually needs to have a very high fidelity of the rocks themselves and kind of like the surface texture so some of the things from my experimenting with some NeRFs there is some more like blobby-ness into it and like how it does from the averaging I think again as you mentioned relates to the priors based on what it's assuming is going to see so I guess would the answer kind of like you said be maybe training it on a bunch of maybe depth maps of the corresponding fields and kind of trying to get the right levels? Would it be using maybe multimodal like reconstruction of like infrared scans out of the same aerial skin would that be a way to kind of direct it to you think or I don't know that's more of a question in that space?
Matt Tancik: Yeah I think there's a lot of different directions that you could go to try to solve some
of the issues you end up seeing in those types of reconstructions. As you mentioned if you have depth data, you could use that to force it to add geometry roughly around where those depths are. You have to be a little bit careful about that for like a normal NeRF reconstruction having to update it typically hurts because if you have enough images, the images are in a way more accurate than what a depth model will always give you because of reflections and other effects. That being said, if you're in a situation where you're very high up in the air and you're looking down or if you just have a few images, then depth can actually be very helpful to remove potential solutions that are clearly wrong. So yeah I think it does depend on the situation.
Student: I come from the industrial sector and for us any kind of reconstruction of a factory is based it's just a nightmare. If there's any other message we have a lot of shiny reflected surfaces everything basically there's shiny and reflective lots of details, cables, transparent surfaces details of interior who knows what it's impossible and the worker that you guys created that you didn't do it it just...very fantastic.
Matt Tancik: I mean compared to photogrammetry which doesn't represent reflections it's obviously a big step up, but I think there has been a lot of interesting work in the last year or two that more explicitly models the reflections to get even better geometry for reflective objects so the there's still a lot of room to improve in that area.
Student: For example in the apartment reality territory it helped us to suddenly be able to track objects that are otherwise you can't capture them put it breaking them correctly and know if this works we can track objects.
Matt Tancik: okay I'll continue on and I can ask for more questions later. So oh yeah it's worth noting that this is very much a group effort. This is one of the more recent photos we've taken of the the NeRF team at or the nerfstudio team at UC Berkeley. Composed of a number of undergrads, Master students, and also PhD students.
So going all the way back to the beginning of the talk, there's this evolution of 2D images. Photorealistic paintings. Now you've got photographs. What's next? In a way we're sort of seeing that evolution happening right now, which is you can take a photograph but if the thing you want in your mind, the realistic thing object that you care about doesn't exist, what do you do? You're kind of out of luck unless you've got some studio to set up a posed bunny in a costume to look like it's in space, it's just not really practical and so that's where these new models that like the stability folks were just talking about are really powerful because you can just ask whatever you want and it'll give you something. Alternatively if you want to modify existing images to for example look like Vincent Van Gogh painting. A few years ago, what would you do you'd have to be really good at Photoshop there'd be a lot of technical skill required to do that, but now we've got all these style transfer tools and other machine learning tools that now I just need the idea and it'll execute for me. So this is what's happening in a 2D space I think it's natural to ask like what about the 3D?
The space is moving pretty quickly there's got to be some progress there and sure enough there is. So Dream Fusion, this is from a group at Google from last year where I can now just add ask a test prompt for example I don't know raccoon wizard and it'll give me a 3D model of a raccoon wizard. So we're making progress there and that progress is happening rather quickly only a few months later Meta released their 3D generative model that also has motion embedded in it. And all these models are ultimately still like NeRF based where the way they work is by sort of it's not exactly this but like they're generating training images using these 2D diffusion models. So this kind of goes to the question that was asked earlier like where do you incorporate these diffusion models so in this case you're doing it from the very beginning because you have nothing to work from you start with text you start creating these images and then you start training these models off these images in practice.
There's not exactly that path but it's roughly that one thing we were interested in is well what happens if you already have something that you want to start with but then you just want to edit it? And so this is where the Instruct-NeRF2NeRF idea originated, where we have a NeRF already. In this case the NeRF was made in nerfstudio and we want to modify it, so I'll just let this play for a few seconds just to show a few different examples. But if you think about what you would have had to do just a few years ago to accomplish this goal, it would have taken a significant amount of effort right. There's you'd have to load it into Blender, retexture it, re-mesh it, but now with simple text you can start getting these effects.
How does it work at a very high level? We render out the scene for each rendered image we feed it into this diffusion model with some text prompt of how we want to modify the image and then use that to create a modified version of this image, which we then set to be like we replace the old data set image with this new data set image so in a way we're updating this data set over time where we update one image at a time every fixed set of iterations and we just repeat this over and over. So for example this is the initial data set and then as the training progresses this data set gets updated so that the NeRF that's being trained is also being updated at the same time. And since these updates are a little bit at a time, it's able to maintain a 3D consistency. And so once we've done that, we can take a NeRF for example, this one of from inside a volcano at Hawaii and we can restyle it. We can make it sunset or we can do even bigger changes like turning it into a desert we can make it stormy. Make it look like it just snowed. We can take a NeRF of a person and put different costumes on them or change their materials. Or since we're at Berkeley there's bear statues all over so we can turn these statues into actual bears.
And sort of this visualizes how this training progresses it starts with the normal NeRF and then each step in the case on the left it becomes snowier and snowier or in the case on the right the person starts to look more and more like Elf and I think we're just sort of scratching the surface of what's possible with these LLM type instruction models with these 3D representations. I imagine in the future, being able to very selectively like click a person and transform them to whatever you want them to be or maybe select the neck of a giraffe and make it longer. These operations that if you think about how you would traditionally do them would involve a significant amount of effort, but describing them is rather easy and as we build these tools better and better, they should be able to just work with word descriptions alone.
So everything I've talked about so far in this presentation has been very art focused where what we care about is the RGB outputs, whether it's for rendering a video or creating a video game asset. But, NeRFs ultimately are a 3D representation and 3D representations are a lot more broad.
And so this kind of I alluded to this in a previous question which is can we have it learn something more than just RGB? And in this case we worked on this recent project called Language Embedded Radiance Fields where we try to embed language into the actual NeRFs so that it's not just learning RGB and color. And then you can query these NeRFs with just standard text. And so there's a variety of different applications. I mean you can obviously just do sort of a hide and seek type application here, but if you were to pair this with robotics with other HCI type applications, you now have like a description of the 3D scene not just a visualization of the 3D scene. I think with like Chat GPT, there's lots of exciting opportunities here. Where you can actually have ChatGPT interact with its scene because it's using the common language of in this case English and again this is based on nerfstudio where you can go ahead and try this now you can type in whatever you want and it'll highlight where it finds those objects in the scene.
And so at a very high level what we do is we take NeRF we combine it with clip which is this model that combines language and images and this allows us to do these reconstructions. And so as a quick example of some of the things that you can query. You can do very long tail objects. Paper filters in a bag. Waldo. Cookbooks. things that you wouldn't normally find in computer vision data sets. You can query at multiple scales. If I create utensils it highlights everything but if I'm specific and ask for The Wooden Spoon it'll highlight The Wooden Spoon I can ask for a spice rack or I can specifically ask for the tomato powder.
I can query visual properties if I ask for yellow it'll highlight all the scenes the the stuff in the scene that is yellow. In this case, the countertop. I can also do abstract queries. If I ask for electricity, it highlights the electrical outlets and also the plugs that are being plugged into the electrical outlet. It can even read text. in this case it's querying "Boops" mug. And there's one mug that has that word on it and that's the one that's most highlighted.
So I'm not going to go into how the method actually works but at a very high level I think it's kind of interesting to think about how you embed this extra information into these scenes. So NeRFs typically just work off of having RGB channels that's your training data, but there's nothing stopping you from just having additional channels. In this case it's clip features which is where we get that language information, but it could really be anything it could be segmentation masks, it could be infrared. But ultimately it gets aggregated in 3D so that you can explore whatever feature you're interested in in 3D.
And so I'll play a couple more videos here's the case where it's doing rather long tail queries.
I really like this one. So this is a bookstore so you can query books which give you all the books but then you can actually query for the specific titles or authors of books and then it'll highlight in the scene where you can actually find that book. So I don't know if it's useful in this application but like you can imagine in grocery store you could ask for your the branded tomato sauce you want and it'll tell you where in the scene you can find that tomato sauce and since it is a 3D representation you could have a reconstruction of say the entire grocery store and it could navigate you directly to where the Heinz Ketchup is three aisles over because it can all be stored in this 3D representation.
Okay so that's language embedded radiance fields and I think this is an area that's going to become more and more interesting as we go especially as we start tying these NeRFs into other systems rather than just using them as a means to create visual outputs. And I just want to very briefly end on sort of like where we're at now. So NeRFs came out again two and a half three years ago and over the last six months to a year, we're starting to see it actually show up in industrial applications both from big companies and also from startups.
So for example Google. Who just sort of quietly announced last week that if you start searching for shoes in Google Shopping, some of the products will be NeRFs that you can look at these 3D objects. Additionally for some restaurants and places in Google Maps, you can actually explore the insides of these locations which are ultimately NeRFs. And then you have startups like Luma that are making more commercial products for things like embedding NeRFs into Unreal Engine. That's what's being shown on the left. Or these text to 3D type applications and generative applications as shown on the right.
So I'll summarize there I don't know how much time we have left but I'm happy to answer questions about any of those last things I just discussed or NeRFs in general.
Professor: Really mind-blowing. I mean you if it's three years old, yeah? it's a kind of like you you're wrapping up 20 years.
Student:
I have one question and then we can go to online questions. When you're projecting to the future, I've seen some work where it allows to you know edit objects out of the scene put them somewhere else eventually probably all of that is on the 2D image space in some way and so on, but for a long time there was like these ideas about boxwood engines. Startups came and failed and so on and I think to London because there's Minecraft, but could you imagine a future where interactive environments are based on NeRF technology?
Matt Tancik: Yeah I think it's a good question. Which I feel like the answer has changed a lot over the last year even. Where initially I would have said no. NeRFs provided a very easy way to optimize and generate this content, but then when it actually came to deploying it converting it to a mesh or one of these more traditional representations seemed like the smarter approach because there has been 20 30 years of optimization and hardware designed specifically for that type of representation.
In the last year or so, we're starting to see more and more players like NVIDIA starting to create hardware more optimized for these neural-based representations. So it's becoming more practical to maybe actually use these in real time applications. So for example like just having it in a game engine that you can move around and explore in, that was impossible a year ago and now it's possible and you can people are using. It it's not quite real time enough to like load it up onto an Xbox, but it's good enough that people in VFX industries are comfortable using it. And then on the flip side, the technology is changing when I describe it as this neural network that takes in a position and produces a color, in reality it's a bit more complicated where nowadays it's really a neural network but it's a whole bunch of them arranged effectively in a voxel grid and that gives you some of the computational efficiencies in terms of processing that voxel grid gives you while also being able to represent these high resolutions. So I think these algorithms will continue to evolve too and especially as they maybe become more and more volumetric with these voxel grids we may see a resurgence of these previous methods that were like game engines based off of voxel grids I think in the past there wasn't a strong enough need to go that direction which is why that area sort of fizzled out, but if they're really closely tied to these like super photorealistic outputs that may be more and more prevalent than maybe they start to re-emerge.
Professor: But if I understand that right so if they you can use something that looks like a chessboard of of NeRFs and you put them together on paper what you look at you can optimize more for what what actually to be confused so it renders process, so from that perspective you could also think actually individual objects or assemblies of objects no other parts like when you come from like parametric designers, so all the individual pieces each of them would be represented by a NeRF and through that super composed them. Which is a really interesting perspective. So in the end of the day then you basically have a scene graph of NeRFs instead of a scene graph of polygons that you could put it into into an interactive environment.
Matt Tancik: Yeah I think maybe two comments on that so like one a lot of research does start splitting things up into separate NeRFs where if you know there's a person walking the scene you have a dynamic NeRF representation for the purpose and but then the background of the scene is a separate NeRF so people are already doing that for just like getting higher quality in the reconstructions. And the second thing is these NeRFs can coexist with traditional graphics. Ultimately it is just a 3D object in space so you could just add a 3D mesh inside a NeRF and so then in that case maybe NeRFs makes sense for representing the background where you want it to be photorealistic but you don't really care about collisions or something like that and then you have normal meshes for foreground objects or vice versa I think we'll just see how where people find their case most useful.
Student: Yeah Matt there's an online question in Q&A. I can also just read it out for the audience. So Jared asked is there any work being done to isolate or externalize reflection information so that reflections could come from the host digital scene rather than the original environment? And I think maybe it's also to add on to that in addition to reflections things like shadow which implicitly gives off information that's maybe not seen from just a camera capture maybe you can extract more semantic information from these you know things like shadow and reflections.
Matt Tancik: Yeah short answer is yeah people are definitely looking into the space where again instead of parameterizing as just a density and a color, you parameterize as a a brdf, bi-directional reflectance field, which is what most things in normal computer gpaphics parameterize as. The one tricky part though is once you start parameterizing it with these more parametric models, you now also have to optimize for the environment lighting. And then potentially as you're doing this optimization of the NeRF you're having to bounce around multiple rays and it becomes a bit more computationally complex. So that's really where the challenge exists at the moment. Is how do you do these breakdowns while still being efficient enough to be practical.
Student: A quick one sorry I know it's 5 p.m I was just reminded that we had a speaker from Meta talking about generating photorealistic avatars and you know it was it took a lot of effort because they had to build this whole dome that's a bunch of cameras capturing multiple different angles and NeRF could be you know a way to alleviate some of the compute that's required but of course you mentioned the scene used to be relatively stable and you kind of have to know exactly what a camera is I don't know if you could speculate sort of moving forward is there like a easier faster way for those kind of things to happen instead of having to you know go into a dome to get your face scanned.
Matt Tancik: Yeah. It really just depends on if you can figure out the priors that describe the 3D World then you need less of this additional information so like in The Meta case they were really trying to I believe they even like changed the lighting so that they can capture the people under different lighting and get every possible representation of the person so that they can make the highest quality reconstruction possible. I think as our priors get better that'll be less and less necessary and it also depends on like what level of quality do you actually need. Already what you could do is just hold a phone out in front of you swipe it around a little bit and create a 3D version of your face with a NeRF. There's a project called Nerfies that's already a few years old and those reconstructions are quite good. Now what those reconstructions don't let you do is like animate the face have it talk relight it and so that's where you need these additional priors which in the Meta case they get by training on more data but there are other research papers that that try to do this more algorithmically.
Professor: 5pm thank you so much that was a very impressive presentation that in my opinion I think everyone in the class as well.
Matt Tancik: yeah thanks for the invite.